
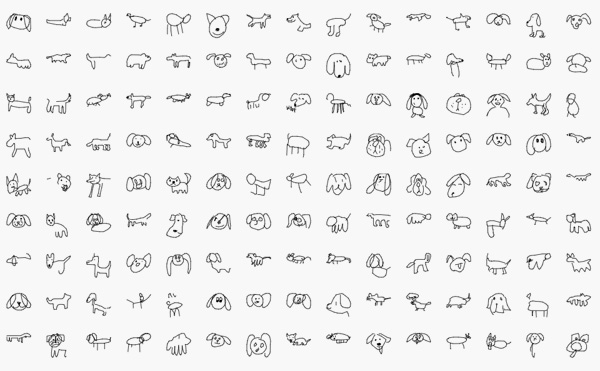
You can also zoom in and see details of each individual drawing, allowing you to dive deeper into single data points. At a glance, you can see proportions of country representations. You, too, can filter for for features such as “random faces” in a 10-country view, which can then be expanded to 100 countries.
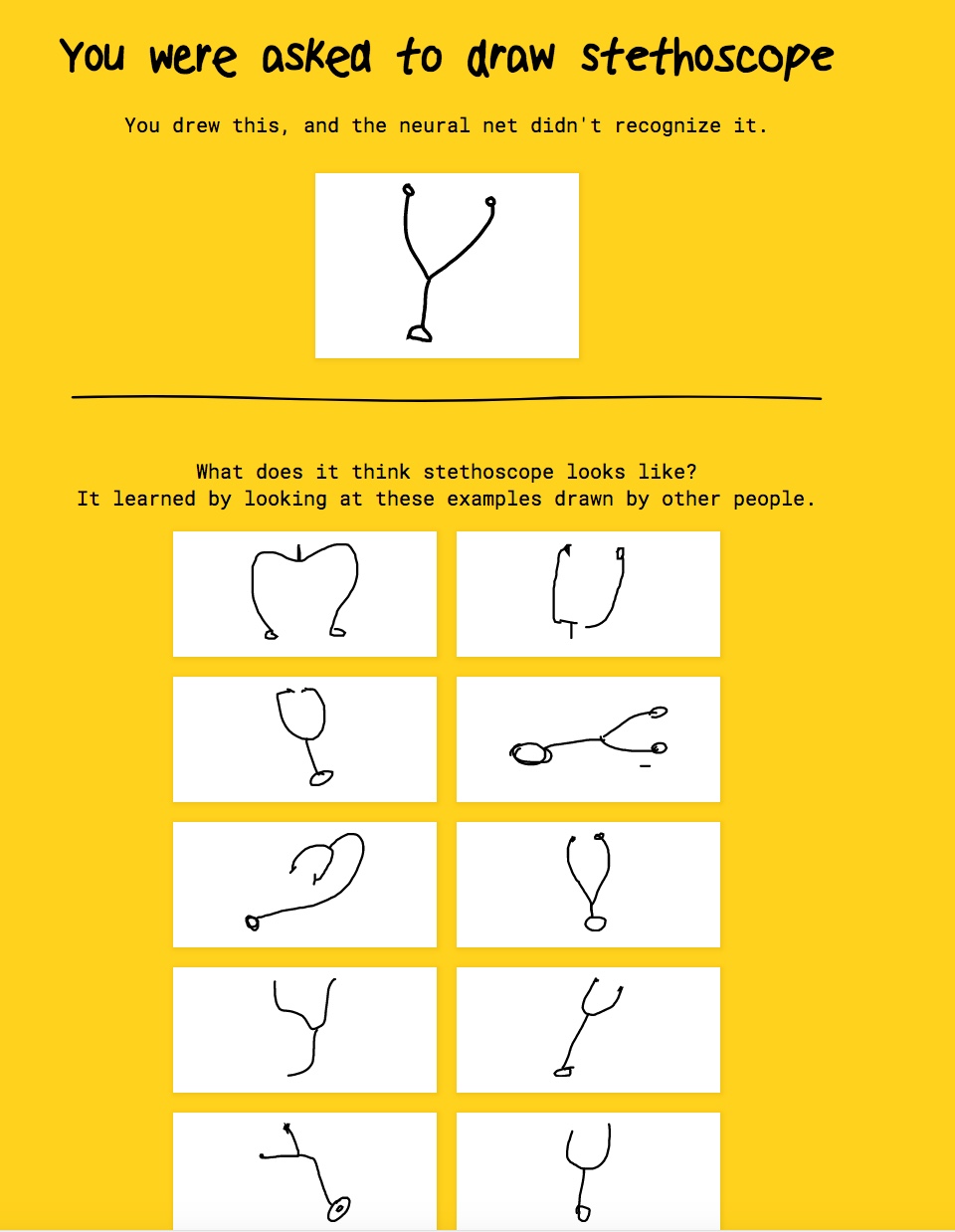
The tool helped us position thousands of drawings by "faceting" them in multiple dimensions by their feature values, such as country, up to 100 countries. Here’s a screenshot from the Quick,Draw! dataset within the Facets tool. The goal is to efficiently, and visually, diagnose how representative large datasets, like the Quick, Draw! Dataset, may be. With the open source tool Facets, released last month as part of Google’s PAIR initiative, one can see patterns across a large dataset quickly. We asked, how can we consistently and efficiently analyze datasets for clues that could point toward latent bias? And what would happen if a team built a classifier based on a non-varied set of data? Because it was so frequently drawn, the neural network learned to recognize only this style as a “shoe.”īut just as in the physical world, in the realm of training data, one size does not fit all.

For example, when we analyzed 115,000+ drawings of shoes in the Quick, Draw! dataset, we discovered that a single style of shoe, which resembles a sneaker, was overwhelmingly represented.
#GOOGLE QUICKDRAW HOW TO#
Overlaying the images also revealed how to improve how we train neural networks when we lack a variety of data - even within a large, open, and international data set. For example, a more straightforward, head-on view was more consistent in some nations side angles in others. These composite drawings, we realized, could reveal how perspectives and preferences differ between audiences from different regions, from the type of bread used in sandwiches to the shape of a coffee cup, to the aesthetic of how to depict objects so they are visually appealing. It’s a considerable amount of data and it’s also a fascinating lens into how to engage a wide variety of people to participate in (1) training machine learning systems, no matter what their technical background and (2) the creation of open data sets that reflect a wide spectrum of cultures and points of view. The dataset currently includes 50 million drawings Quick Draw! players have generated (we will continue to release more of the 800 million drawings over time). to South Africa.Īnd now we are releasing an open dataset based on these drawings so that people around the world can contribute to, analyze, and inform product design with this data. While the goal of Quick, Draw! was simply to create a fun game that runs on machine learning, it has resulted in 800 million drawings from twenty million people in 100 nations, from Brazil to Japan to the U.S. The system will try to guess what their drawing depicts, within 20 seconds. A group of Googlers designed Quick, Draw! as a way for anyone to interact with a machine learning system in a fun way, drawing everyday objects like trees and mugs. Over the last six months, we’ve seen such a dataset emerge from users of Quick, Draw!, Google’s latest approach to helping wide, international audiences understand how neural networks work. In order to train these machine learning systems, open, global - and growing - datasets are needed. As such, researchers and designers seeking to create products that are useful and accessible for everyone often face the challenge of finding data sets that reflect the variety and backgrounds of users around the world.
#GOOGLE QUICKDRAW SOFTWARE#
Machine learning systems are increasingly influencing many aspects of everyday life, and are used by both the hardware and software products that serve people globally. Posted by Reena Jana, Creative Lead, Business Inclusion, and Josh Lovejoy, UX Designer, Google Research
